The AI-ready Automation Partner
way to turn AI into business performance.
Value Added Tech.
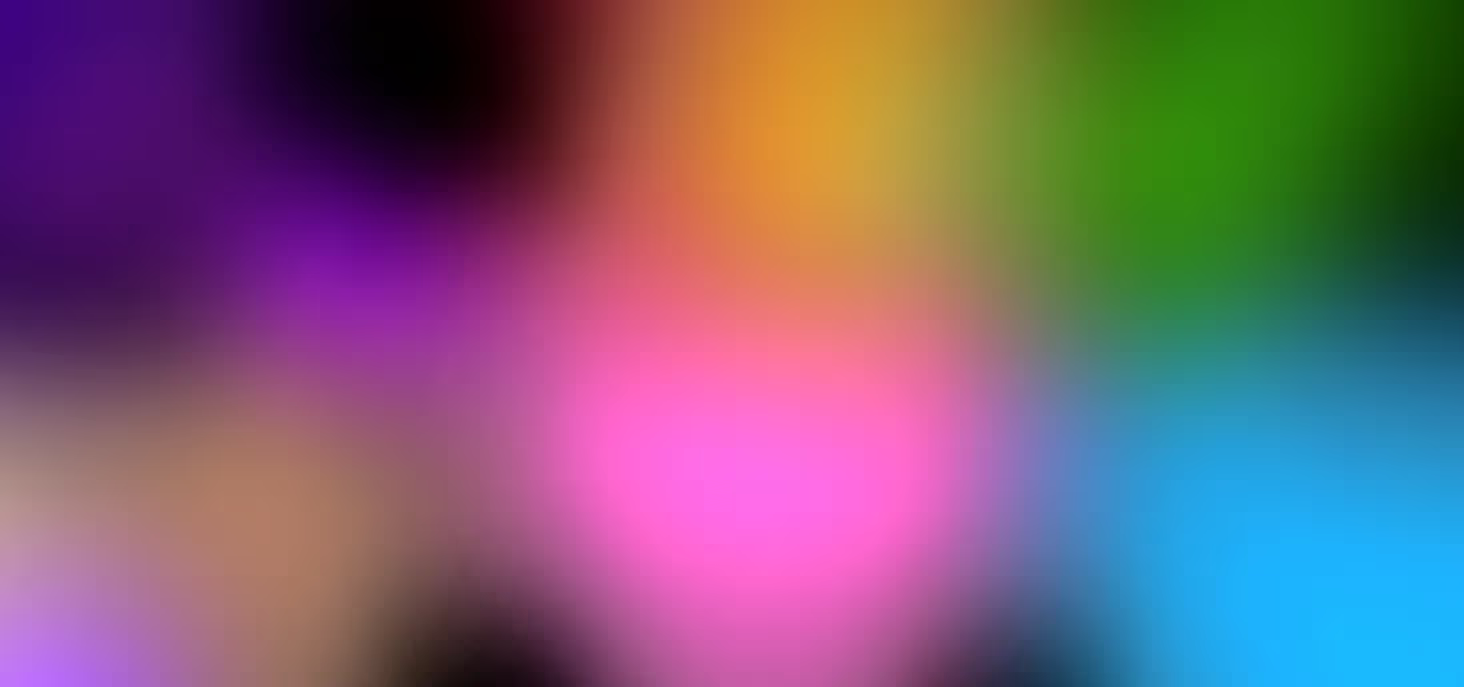
Beyond AI Buzzwords: Measurable Business Value
Where practical AI automation drives ROI, not trendy technology promises
Effective AI automation prioritizes tangible business outcomes over flashy features. When implemented strategically, these solutions solve real operational challenges and deliver measurable performance improvements across your organization.
Practical Applications Across Industries
Revenue Generation & Sales Acceleration
Properly implemented AI shortens sales cycles by 28% by prioritizing high-potential prospects and recommending next-best actions for sales teams. Companies report 35% higher conversion rates and 24% larger average deal sizes when replacing intuition-based selling with data-driven engagement strategies.
Operational Efficiency & Cost Reduction
AI implementation in core operations reduces process handling times by up to 65% while eliminating 73% of manual errors. This translates to average cost savings of 28-42% in transaction-heavy business functions while improving compliance and consistency across operations.
Supply Chain & Inventory Optimization
AI implementation reduces inventory carrying costs by 32% while simultaneously decreasing stockouts by 47%. This dual optimization solves the traditional tradeoff between capital efficiency and product availability, improving both financial performance and customer satisfaction.
Marketing Effectiveness & Customer Acquisition
AI transforms marketing from campaign-based to continuous optimization, reducing customer acquisition costs by 31% while improving targeting precision. Organizations implementing AI-driven marketing report 43% higher ROI on advertising spend and 37% improvement in qualified lead generation.
Customer Retention & Lifetime Value
AI enables proactive customer experience management, identifying satisfaction issues before they lead to churn. Organizations report 36% improved retention rates and 27% increased customer lifetime value through early intervention systems and personalized engagement capabilities.
Ready for Practical AI Solutions?
Discover how strategic automation can deliver measurable value and tangible ROI for your business operations.
Request Value AssessmentExceptional partnership
AI-First Business Process Automation
We integrate AI technology directly into your business processes. Our solutions create systems that improve automatically over time. We eliminate repetitive tasks while giving your team powerful insights and smart decision-making tools to work more effectively.
Specializing in sales pipelines, marketing campaigns, HR processes, customer service, and financial operations automation.
Seamless Integration
Achieve tangible results through strategic automation. Cut manual data entry by 60-80%. Boost team productivity by 40-50%. Reduce acquisition costs by 25-35%. Our methodology ensures solutions perfectly aligned with your unique business challenges and growth objectives.
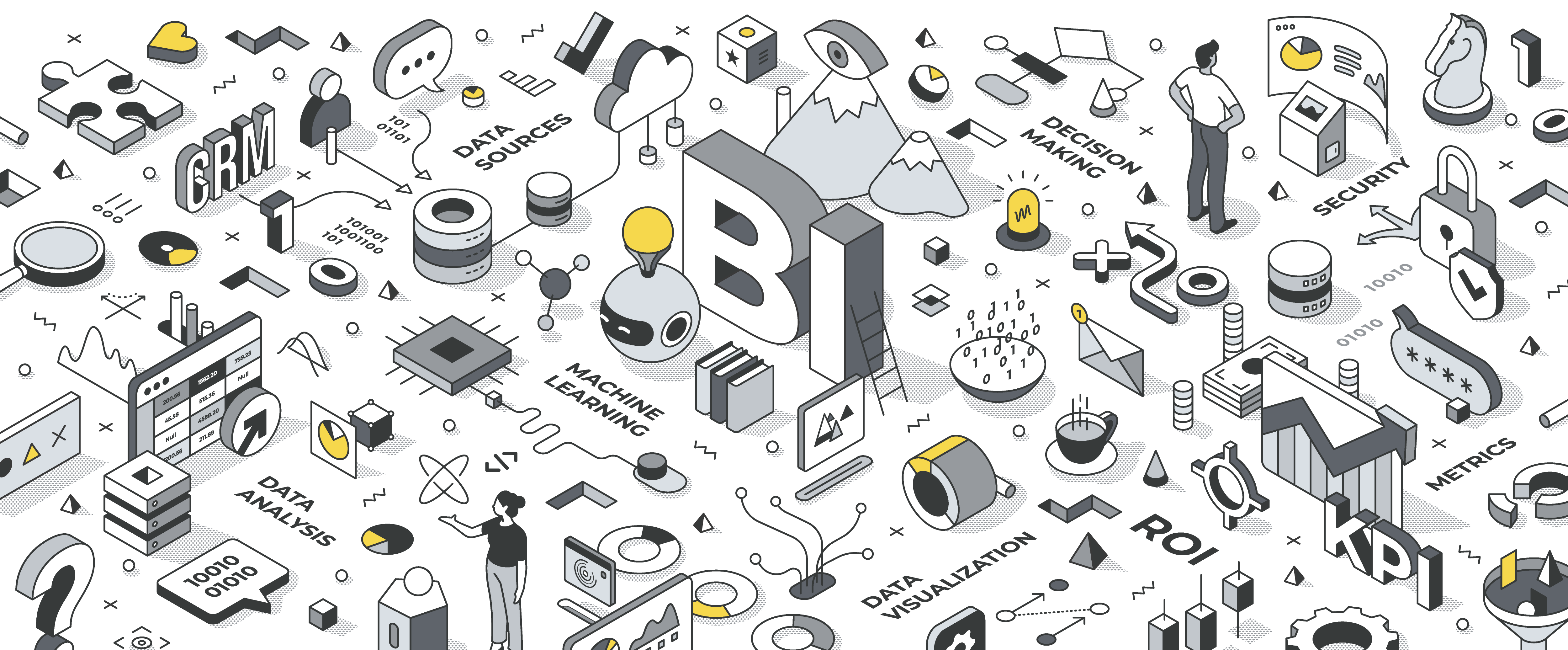
Scalable Growth
Integrate AI seamlessly into existing processes without disruption. Scale intelligent workflows that enhance human decision-making. Bridge the gap between cutting-edge technology and practical business applications with flexible architectures designed to evolve with emerging technologies.
Best-in-Class Technology
Leverage the industry's most powerful platforms including make.com, AWS, OpenAI, and Anthropic through our specialized expertise. As a make.com Gold Partner, we implement automation solutions using only the most innovative and reliable technologies. Transform your business processes with tools specifically selected to deliver maximum impact for your unique operational challenges.
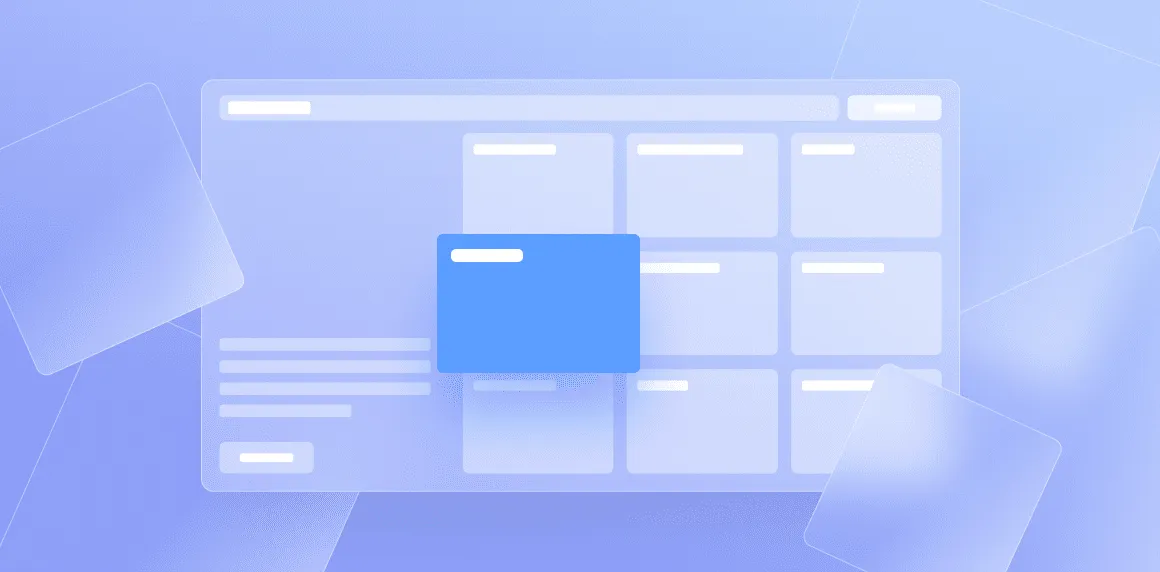
Essential resources for your AI journey
Intelligent Automation Revolution: How a Leading Investment Firm Achieved 300% Processing Capacity with AI-Powered Financial Operations
A financial investment firm transformed invoice processing with intelligent automation, integrating OCR, AI, and workflow systems. The solution reduced processing time by 60%, cut errors by 80%, and increased capacity by 300%, enabling accountants to focus on strategic work rather than manual tasks.
KEEP READINGRobusta. Scaling Make.com for Enterprises.
Discover how Value Added Tech addresses challenges in automating business with Make.com, offering innovative solutions like automated scenario monitoring and robust backup systems. Learn about our strategies to maintain development standards and prevent failures caused by updates, ensuring efficient and reliable operations.
AI is moving fast
33% of enterprise software will include agentic AI by 2028. Get Gartner's report to prepare your organization today.
Automating a call center with AI calling agents
See how we helped a US-based marketing agency automate appointment booking for 600 clients, achieving a 60% reduction in operational costs.